Audio diffusion models have achieved high-quality speech, music, and Foley sound synthesis, yet they predominantly excel at sample generation rather than parameter optimization. Tasks like physically informed impact sound generation or prompt-driven source separation require models that can adjust explicit, interpretable parameters under structural constraints. Score Distillation Sampling (SDS)—which has powered text-to-3D and image editing by backpropagating through pretrained diffusion priors—has not yet been applied to audio. Adapting SDS to audio diffusion allows optimizing parametric audio representations without assembling large task-specific datasets, bridging modern generative models with parameterized synthesis workflows.
Classic audio techniques—such as frequency modulation (FM) synthesis, which uses operator-modulated oscillators to craft rich timbres, and physically grounded impact-sound simulators—provide compact, interpretable parameter spaces. Similarly, source separation has evolved from matrix factorization to neural and text-guided methods for isolating components like vocals or instruments. By integrating SDS updates with pretrained audio diffusion models, one can leverage learned generative priors to guide the optimization of FM parameters, impact-sound simulators, or separation masks directly from high-level prompts, uniting signal-processing interpretability with the flexibility of modern diffusion-based generation.
Researchers from NVIDIA and MIT introduce Audio-SDS, an extension of SDS for text-conditioned audio diffusion models. Audio-SDS leverages a single pretrained model to perform various audio tasks without requiring specialized datasets. Distilling generative priors into parametric audio representations facilitates tasks like impact sound simulation, FM synthesis parameter calibration, and source separation. The framework combines data-driven priors with explicit parameter control, producing perceptually convincing results. Key improvements include a stable decoder-based SDS, multistep denoising, and a multiscale spectrogram approach for better high-frequency detail and realism.
The study discusses applying SDS to audio diffusion models. Inspired by DreamFusion, SDS generates stereo audio through a rendering function, improving performance by bypassing encoder gradients and focusing instead on the decoded audio. The methodology is enhanced by three modifications: avoiding encoder instability, emphasizing spectrogram features to highlight high-frequency details, and using multi-step denoising for better stability. Applications of Audio-SDS include FM synthesizers, impact sound synthesis, and source separation. These tasks show how SDS adapts to different audio domains without retraining, ensuring that synthesized audio aligns with textual prompts while maintaining high fidelity.
The performance of the Audio-SDS framework is demonstrated across three tasks: FM synthesis, impact synthesis, and source separation. The experiments are designed to test the framework’s effectiveness using both subjective (listening tests) and objective metrics such as the CLAP score, distance to ground truth, and Signal-to-Distortion Ratio (SDR). Pretrained models, such as the Stable Audio Open checkpoint, are used for these tasks. The results show significant audio synthesis and separation improvements, with clear alignment to text prompts.
In conclusion, the study introduces Audio-SDS, a method that extends SDS to text-conditioned audio diffusion models. Using a single pretrained model, Audio-SDS enables a variety of tasks, such as simulating physically informed impact sounds, adjusting FM synthesis parameters, and performing source separation based on prompts. The approach unifies data-driven priors with user-defined representations, eliminating the need for large, domain-specific datasets. While there are challenges in model coverage, latent encoding artifacts, and optimization sensitivity, Audio-SDS demonstrates the potential of distillation-based methods for multimodal research, particularly in audio-related tasks.
Check out the Paper and Project Page. All credit for this research goes to the researchers of this project. Also, feel free to follow us on Twitter and don’t forget to join our 90k+ ML SubReddit.
Here’s a brief overview of what we’re building at Marktechpost:
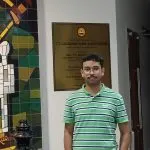
Sana Hassan, a consulting intern at Marktechpost and dual-degree student at IIT Madras, is passionate about applying technology and AI to address real-world challenges. With a keen interest in solving practical problems, he brings a fresh perspective to the intersection of AI and real-life solutions.