Recent advancements in LLMs such as OpenAI-o1, DeepSeek-R1, and Kimi-1.5 have significantly improved their performance on complex mathematical reasoning tasks. Reinforcement Learning with Verifiable Reward (RLVR) is a key contributor to these improvements, which uses rule-based rewards, typically a binary signal indicating whether a model’s solution to a problem is correct. Beyond enhancing final output accuracy, RLVR has also been observed to foster beneficial cognitive behaviors like self-reflection and improve generalization across tasks. While much research has focused on optimizing reinforcement learning algorithms like PPO and GRPO for greater stability and performance, the influence of training data—its quantity and quality—remains less understood. Questions around how much and what kind of data is truly effective for RLVR are still open, despite some work like LIMR introducing metrics to identify impactful examples and reduce dataset size while maintaining performance.
In contrast to the extensive research on data selection in supervised fine-tuning and human feedback-based reinforcement learning, the role of data in RLVR has seen limited exploration. While LIMR demonstrated that using a small subset of data (1.4k out of 8.5k examples) could maintain performance, it did not examine the extreme case of minimal data use. Another concurrent study found that even training with just four PPO examples led to notable improvements, but this finding wasn’t deeply investigated or benchmarked against full-dataset performance. Although RLVR shows great promise for enhancing reasoning in LLMs, a deeper, systematic study of data efficiency and selection in this context is still lacking.
Researchers from the University of Washington, University of Southern California, Microsoft, University of California, Santa Cruz, and Georgia Institute of Technology show that RLVR can significantly enhance large language models’ mathematical reasoning using a single training example, 1-shot RLVR. Applying it to Qwen2.5-Math-1.5B improves its MATH500 accuracy from 36.0% to 73.6%, matching the performance of much larger datasets. The improvements generalize across models, tasks, and algorithms. The study also reveals effects like cross-domain generalization, increased self-reflection, and post-saturation generalization, and highlights the roles of policy gradient loss and entropy-driven exploration.
The study investigates how much the RLVR training dataset can be reduced while retaining comparable performance to the full dataset. Remarkably, the authors find that a single training example—1-shot RLVR—can significantly boost mathematical reasoning in LLMs. The study shows that this effect generalizes across tasks, models, and domains. Interestingly, training on one example often enhances performance on unrelated domains. A simple data selection strategy based on training accuracy variance is proposed, but results show that even randomly chosen examples can yield major gains.
The study evaluates their method using Qwen2.5-Math-1.5B as the primary model and other models like Qwen2.5-Math-7B, Llama-3.2-3 B-Instructt, and DeepSeek-R1-DistillQwen-1.5 BB. They use a 1,209-example subset of the DeepScaleR dataset for data selection, and the MATH dataset for comparison. Training involves the Verl pipeline, with carefully chosen hyperparameters and batch configurations. Surprisingly, training with just one or two examples—especially π1 and π13—leads to strong generalization, even beyond math tasks. This “post-saturation generalization” persists despite overfitting signs. The study also finds increased model self-reflection and shows that even simple examples can significantly enhance performance across domains.
In conclusion, the study explores the mechanisms behind the success of 1-shot RLVR, demonstrating that base models already possess strong reasoning abilities. Experiments show that even a single example can significantly improve performance on reasoning tasks, suggesting the model’s inherent capacity for reasoning. The study highlights that policy gradient loss is key to 1-shot RLVR’s effectiveness, with entropy loss further enhancing performance. Additionally, encouraging exploration through techniques like entropy regularization can improve post-saturation generalization. The findings also emphasize the need for careful data selection to optimize the model’s performance, particularly in data-constrained scenarios.
Check out the Paper and GitHub Page. Also, don’t forget to follow us on Twitter and join our Telegram Channel and LinkedIn Group. Don’t Forget to join our 90k+ ML SubReddit.
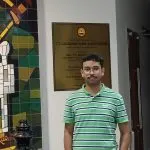
Sana Hassan, a consulting intern at Marktechpost and dual-degree student at IIT Madras, is passionate about applying technology and AI to address real-world challenges. With a keen interest in solving practical problems, he brings a fresh perspective to the intersection of AI and real-life solutions.